Models That Prove Their Own Correctness
Keywords:
]AI Model safety, Self-Proving models, Verification algorithm, Soundness property, interactive proof, bounded probabilistic proof, software validation, software verificationAbstract
How can we trust the correctness of a learned model on a particular input of interest? Model accuracy is typically measured *on average* over a distribution of inputs, giving no guarantee for any fixed input. This paper proposes a theoretically-founded solution to this problem: to train *Self-Proving models* that prove the correctness of their output to a verification algorithm V via an Interactive Proof. Self-Proving models satisfy that, with high probability over a random input, the model generates a correct output *and* successfully proves its correctness to V. The *soundness* property of V guarantees that, for *every* input, no model can convince V of the correctness of an incorrect output. Thus, a Self-Proving model proves correctness of most of its outputs, while *all* incorrect outputs (of any model) are detected by V. We devise a generic method for learning Self-Proving models, and we prove convergence bounds under certain assumptions. The theoretical framework and results are complemented by experiments on an arithmetic capability: computing the greatest common divisor (GCD) of two integers. Our learning method is used to train a Self-Proving transformer that computes the GCD *and* proves the correctness of its answer.
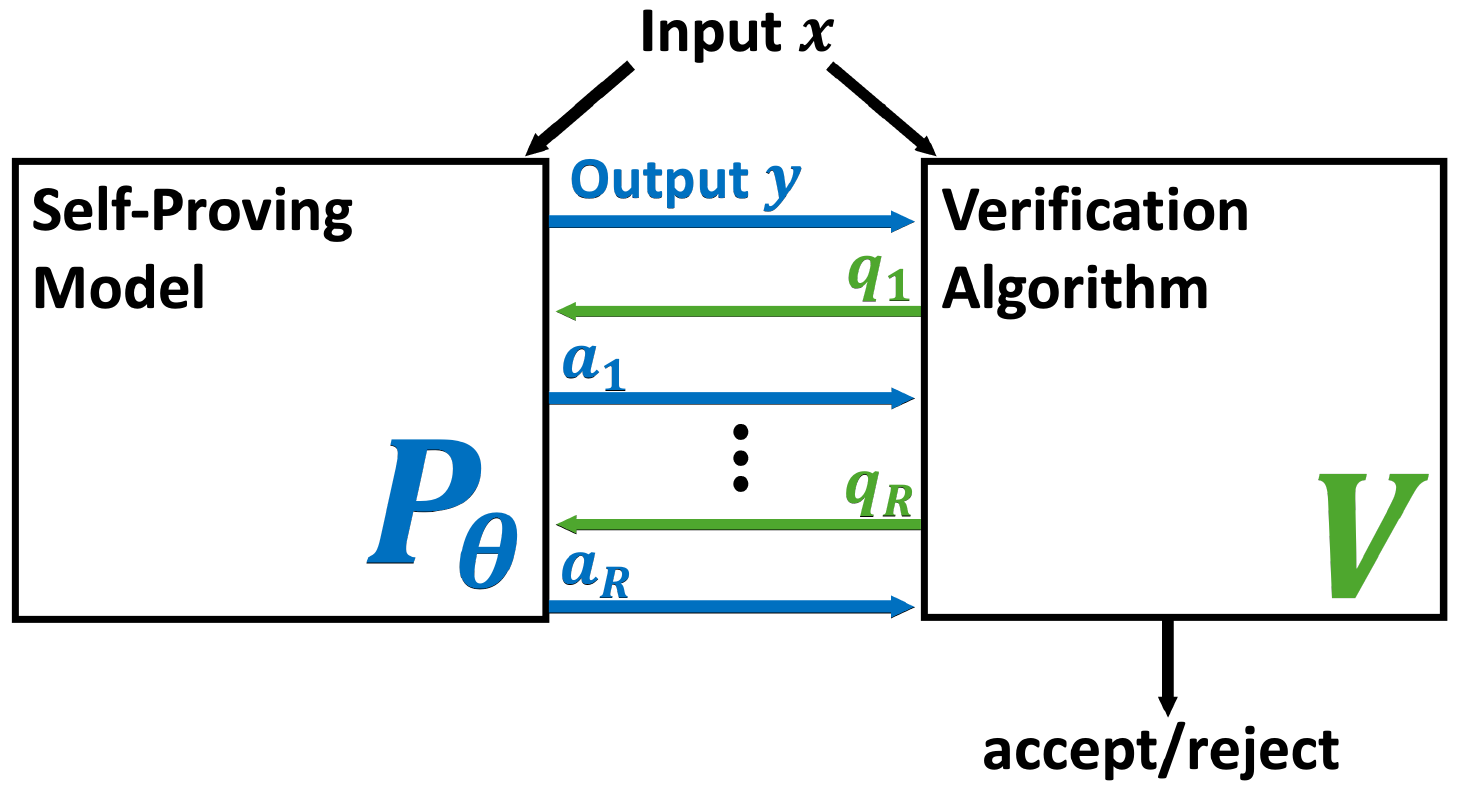
Downloads
Published
How to Cite
Issue
Section
License
Copyright (c) 2024 Orr Paradise

This work is licensed under a Creative Commons Attribution-NoDerivatives 4.0 International License.